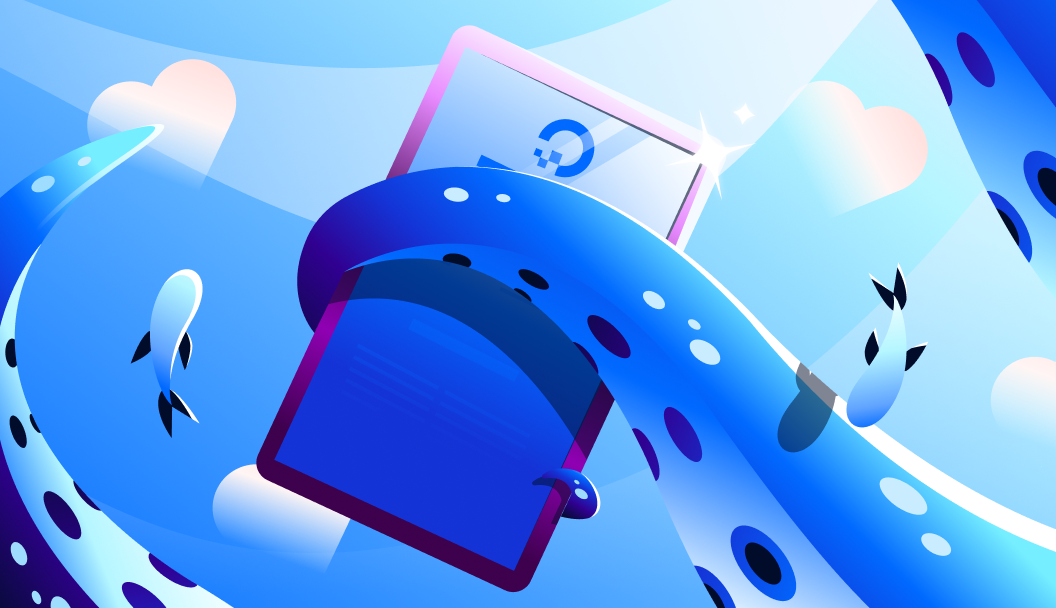
Unlock Business Potential: A Practical Guide to Generative AI in 2024
Generative AI is revolutionizing how businesses operate and create. It’s not just hype; it's a powerful tool that can improve internal processes, enhance product development, and personalize customer experiences. From generating code to crafting marketing campaigns, generative AI models are reshaping industries.
This article will break down what generative AI is, how it works, and the best strategies for integrating it into your business. Discover how to leverage this transformative technology for tangible results.
What Exactly Is Generative AI? Unveiling the Definition
Generative AI refers to AI systems that create original content by learning from existing information. These systems use machine learning models like large language models (LLMs) to create text, images, audio, video, and even synthetic data. By understanding patterns in data, generative AI can produce realistic and innovative outputs.
These models use architectures like transformers, recurrent neural networks, and generative adversarial networks to generate diverse and realistic AI outputs.
How Does Generative AI Work? A Step-by-Step Breakdown
Generative AI works by using machine learning algorithms and large datasets to create new and meaningful outputs. Here's a simplified look at the process:
- Input Data Collection: Data is gathered from various sources like web crawlers, databases, and sensors, then cleaned for consistency.
- AI Model Training: AI models (LLMs, diffusion models, or GANs) learn data patterns and relationships from labeled or unlabeled data.
- Understanding Data Patterns: Generative models analyze inputs and use probability distribution to capture latent representations of the data.
- New Output Generation: The AI system creates content that aligns with user-defined goals, using neural networks like transformers.
- Feedback Refinement: Human feedback or additional AI algorithms refine outputs for better quality and accuracy.
Maximizing Business Benefits: Exploring Generative AI's Potential
Generative AI can simplify content creation, improve data utilization, advance personalized solutions, speed up prototyping, and streamline complex processes.
Here's a closer look at those benefits:
- Simplifies Content Creation: Generative AI produces realistic images, videos, and text, beneficial for media, advertising, and virtual reality.
- Improves Data Utilization: Generative AI generates synthetic data to fill gaps in datasets, enhancing AI model accuracy and compliance.
- Advances Personalized Solutions: Generative AI creates customized outputs based on individual preferences, ideal for marketing and education.
- Speeds Up Prototyping: Generative models simplify prototyping processes for software development and product design.
- Streamlines Complex Processes: Generative AI automates tasks like data analysis and AI-generated content creation, increasing efficiency.
Overcoming Obstacles: Addressing Generative AI Challenges
Like any technology, generative AI tools come with challenges that need to be addressed for optimal results. From data quality to ethical considerations, let's break it down:
- Data Quality Limitations: Incomplete or biased data leads to inaccurate outputs, affecting reliability.
- Computational Complexity: Training generative AI systems requires significant computational resources, impacting scalability.
- Lack of Explainability: The "black box" nature of generative models makes it difficult to understand decision-making processes.
- Ethical and Misuse Risks: Generative AI can be misused to create deepfakes and spread misinformation, raising ethical concerns.
- Generalization Issues: Models can struggle with overfitting or underfitting, reducing performance in real-world applications.
Generative AI Models Explained: A Comprehensive Overview
Generative AI models come in various architectures, each specializing in generating specific content types. Here are some prominent types:
- Generative Adversarial Networks (GANs): Generate realistic images and synthetic data through a competitive process.
- Diffusion Models: Create images and art by gradually adding and reversing noise in data.
- Large Language Models (LLMs): Generate human-like language for NLP tasks and text generation.
- Recurrent Neural Networks (RNNs): Handle sequential data for time-series forecasting and language modeling.
- Variational Autoencoders (VAEs): Compress and reconstruct data to generate new samples and detect anomalies.
- Transformers: Process data in parallel for language modeling, code generation, and machine translation.
Best Practices for Integrating Generative AI into Your Business
Successfully integrating AI content generation into your business requires strategic planning and execution. Here are some best practices:
- Choose the Right Service: Select tools based on cost, API limits, and features aligning with your business needs.
- Improve Prompt Engineering Capabilities: Develop effective prompts to get the desired outputs; generic instructions lead to generic results.
- Establish Usage Guidelines: Create internal policies to ensure consistency, compliance, and data security.
- Monitor Quality and Costs: Track metrics like response relevance and error rates to optimize performance and control spending.
- Ensure Security and Compliance: Use platforms with robust data protection measures and align with industry standards like GDPR.
- Build Integration Workflows: Automate repetitive tasks by integrating generative AI with existing tools and platforms.
Ready to Supercharge Your Applications? Consider DigitalOcean's GenAI Platform
DigitalOcean's new GenAI Platform allows developers to integrate AI agent capabilities into their applications without managing complex infrastructure. With direct access to foundational models from Meta, Mistral AI, and Anthropic, intuitive customization tools, and robust safety features, it simplifies the process of building and deploying sophisticated AI agents. Sign up to get started today!